Israeli researchers develop algorithm to predict infectious diseases
The researchers turned their attention to diagnosing the onset of tuberculosis, caused by bacteria that can hide dormant in the body for years.
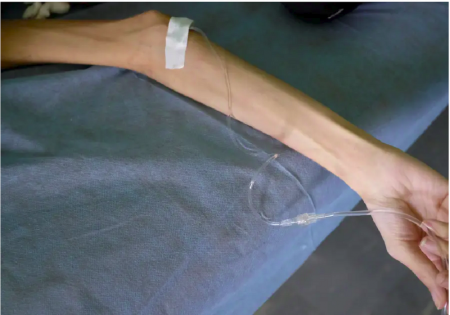
Researchers at the Weizmann Institute of Science claim they have developed an algorithm to predict the onset of infectious diseases, including tuberculosis.
While in certain cases the immune system can kill bacteria and, in other cases, bacteria can overcome immune defenses, there are diseases like tuberculosis where bacteria can lie dormant for years - sometimes causing disease at a later stage and sometimes remaining in hibernation.
Testing a hypothesis as to whether the future development of diseases is determined within the first 24-48 hours after infection, scientists led by Dr. Roi Avraham from the institute’s Biological Regulation Department used a method developed at the institute to sequence gene activity in real meetings between thousands of immune cells and Salmonella bacteria.
Unlike in standard laboratory tests, the method enabled researchers to see the responses of cells to the bacteria and map out activation profiles of each cell. Confirming their hypothesis, researchers identified different responses and patterns from the initial meetings between the cells and bacteria, and their later outcomes.
Building on their single-cell sequencing for Salmonella infection, the researchers developed an algorithm - based on a method known as deconvolution - to extract similar information of individual cell properties from standard blood test data sets.
"The algorithm we developed can not only define the ensemble of immune cells that take part in the response, it can reveal their activity levels and thus the potential strength of the immune response," said Dr. Noa Bossel Ben Moshe, who co-led the research together with Dr. Shelly Hen-Avivi in Avraham's group.
The algorithm was first tested in blood samples taken from healthy people from the Netherlands. Some samples were infected with Salmonella bacteria and the immune response recorded. While existing genomic analysis methods did not uncover differences between groups, the algorithm revealed significant differences that were tied to subsequent variations in bacteria-killing abilities.
The researchers then turned their attention to diagnosing the onset of tuberculosis, caused by bacteria that can hide dormant in the body for years.
Utilizing a British blood test database following patients and carriers for a period of two years – enabling the application of the algorithm to both groups and the subset that shifted from carrier to disease onset during that time – researchers found that the activity levels of immune cells called monocytes could predict the onset or future course of the disease.
"The algorithm is based on the ‘first impressions’ of immune cells and Salmonella, which cause a very different type of illness than mycobacterium tuberculosis," said Hen-Avivi.
"Still, we were able to predict early on which of the carriers would develop the active form of the disease."
While antibiotic resistance poses a major challenge to treating tuberculosis today, the researchers believe their algorithm could boost the success of treatment.
"If those who are at risk of active disease could be identified when the bacterial load is smaller, their chances of recovery will be better," said Avraham.
"And the state medical systems in countries where tuberculosis is endemic might have a better way to keep the suffering and incidence of sickness down while reducing the cost of treatment."
Avraham's team now intends to continue their research, expanding their database on tuberculosis and also other pathogens, to refine their algorithm and develop tools that may be used in the future to predict the development and course of a number of infectious diseases.